Deep Learning Model Developed to Optimize Isotope Production Target Cooling Systems
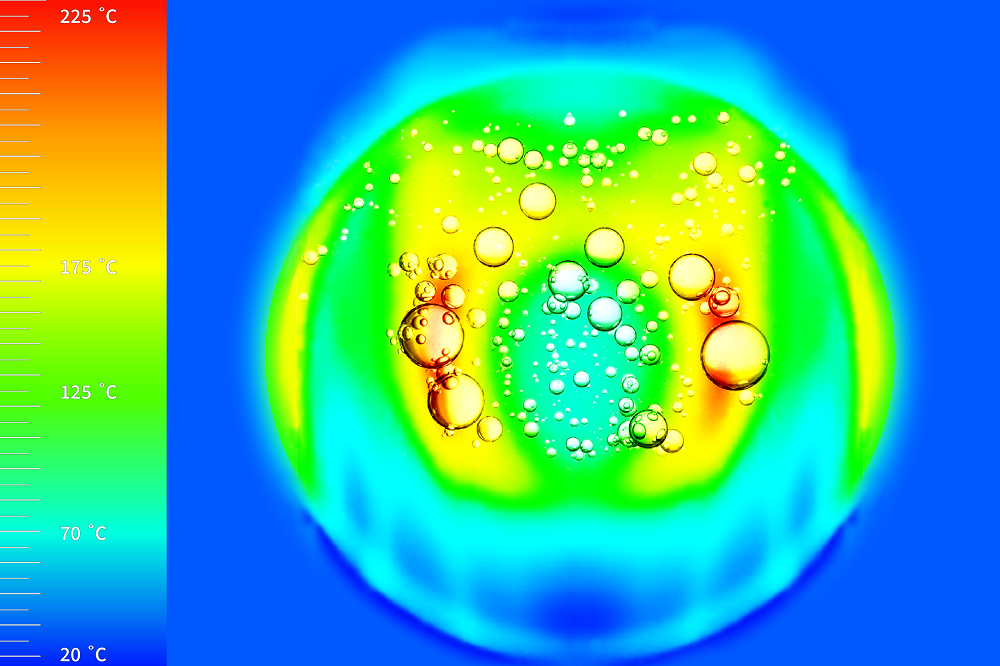
Image courtesy of Los Alamos National Laboratory Abstract representation of subcooled flow boiling modeled on experimental data collected for the Los Alamos National Laboratory Isotope Production Facility.
Particle accelerators and isotope production facilities depend on cooling for the proper function of target systems during irradiation. The target systems at Los Alamos Neutron Science Center’s (LANSCE) Isotope Production Facility rely on a series of water channels in the target system to remove heat as targets are irradiated. The high heat flux between the target surface and the flowing water in the channels likely produces subcooled flow boiling. With concerns about target failures caused by reaching critical heat flux and boiling crisis, researchers wanted to determine maximum operating conditions, but radiation levels present during target irradiation prevent live monitoring. Instead, researchers built an experimental apparatus that mimics the target system to collect temperature data and high-speed video of bubble activity in the mock cooling channel.
Researchers adapted a deep-learning tool that was originally developed to detect cells in biological images. With the adapted algorithm, the team extracted critical bubble parameters from the high-speed video, enabling the researchers to develop and validate a framework to predict a complete boiling curve. The researchers then confirmed that current operations are well below the predicted critical heat flux.
Supported by the Department of Energy Isotope Program managed by the Office of Science for Isotope R&D and Production, the research was conducted at Los Alamos National Laboratory. This pioneering work not only enhances the efficiency and safety at the LANSCE Isotope Production Facility but also potentially benefits other particle accelerator target applications and isotope production facilities that lack in-beam monitoring at different accelerators worldwide.